Reinforcement Learning and Optimal Control
by Dimitri P. Bertsekas
Publisher: Athena Scientific 2019
Number of pages: 276
Description:
The purpose of the book is to consider large and challenging multistage decision problems, which can be solved in principle by dynamic programming and optimal control, but their exact solution is computationally intractable. We discuss solution methods that rely on approximations to produce suboptimal policies with adequate performance.
Download or read it online for free here:
Download link
(multiple PDF files)
Similar books
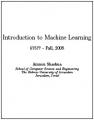
by Amnon Shashua - arXiv
Introduction to Machine learning covering Statistical Inference (Bayes, EM, ML/MaxEnt duality), algebraic and spectral methods (PCA, LDA, CCA, Clustering), and PAC learning (the Formal model, VC dimension, Double Sampling theorem).
(22618 views)
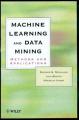
by Aaron Hertzmann - University of Toronto
Contents: Introduction to Machine Learning; Linear Regression; Nonlinear Regression; Quadratics; Basic Probability Theory; Probability Density Functions; Estimation; Classification; Gradient Descent; Cross Validation; Bayesian Methods; and more.
(10272 views)
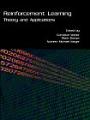
by C. Weber, M. Elshaw, N. M. Mayer - InTech
This book describes and extends the scope of reinforcement learning. It also shows that there is already wide usage in numerous fields. Reinforcement learning can tackle control tasks that are too complex for traditional controllers.
(21614 views)
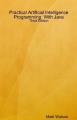
by Mark Watson - Lulu.com
The book uses the author's libraries and the best of open source software to introduce AI (Artificial Intelligence) technologies like neural networks, genetic algorithms, expert systems, machine learning, and NLP (natural language processing).
(25021 views)