Machine Learning: A Probabilistic Perspective
by Kevin Patrick Murphy
Publisher: The MIT Press 2012
ISBN-13: 9780262018029
Number of pages: 1098
Description:
A comprehensive introduction to machine learning that uses probabilistic models and inference as a unifying approach. The book is written in an informal, accessible style, complete with pseudo-code for the most important algorithms.
Download or read it online for free here:
Download link
(46MB, PDF)
Similar books

by Carl E. Rasmussen, Christopher K. I. Williams - The MIT Press
Gaussian processes provide a principled, practical, probabilistic approach to learning in kernel machines. The treatment is comprehensive and self-contained, targeted at researchers and students in machine learning and applied statistics.
(27720 views)
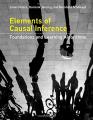
by J. Peters, D. Janzing, B. Schölkopf - The MIT Press
This book offers a self-contained and concise introduction to causal models and how to learn them from data. The book teaches readers how to use causal models: how to compute intervention distributions, how to infer causal models from data ...
(6052 views)
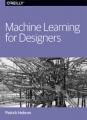
by Patrick Hebron - O'Reilly Media
This book introduces you to contemporary machine learning systems and helps you integrate machine-learning capabilities into your user-facing designs. Patrick Hebron explains how machine-learning applications can affect the way you design websites.
(6752 views)
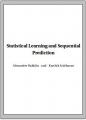
by Alexander Rakhlin, Karthik Sridharan - University of Pennsylvania
This text focuses on theoretical aspects of Statistical Learning and Sequential Prediction. The minimax approach, which we emphasize throughout the course, offers a systematic way of comparing learning problems. We will discuss learning algorithms...
(6615 views)