Reinforcement Learning
by C. Weber, M. Elshaw, N. M. Mayer
Publisher: InTech 2008
ISBN-13: 9783902613141
Number of pages: 424
Description:
The first 11 chapters of this book describe and extend the scope of reinforcement learning. The remaining 11 chapters show that there is already wide usage in numerous fields. Reinforcement learning can tackle control tasks that are too complex for traditional, hand-designed, non-learning controllers. As learning computers can deal with technical complexities, the tasks of human operators remain to specify goals on increasingly higher levels.
Download or read it online for free here:
Download link
(12MB, PDF)
Similar books
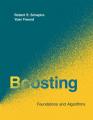
by Robert E. Schapire, Yoav Freund - The MIT Press
Boosting is an approach to machine learning based on the idea of creating a highly accurate predictor by combining many weak and inaccurate 'rules of thumb'. A remarkably rich theory has evolved around boosting, with connections to a range of topics.
(6495 views)
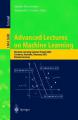
by Alex Smola, S.V.N. Vishwanathan - Cambridge University Press
Over the past two decades Machine Learning has become one of the mainstays of information technology and a rather central part of our life. Smart data analysis will become even more pervasive as a necessary ingredient for technological progress.
(9660 views)
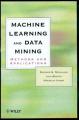
by Aaron Hertzmann - University of Toronto
Contents: Introduction to Machine Learning; Linear Regression; Nonlinear Regression; Quadratics; Basic Probability Theory; Probability Density Functions; Estimation; Classification; Gradient Descent; Cross Validation; Bayesian Methods; and more.
(10007 views)
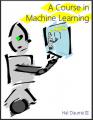
by Hal Daumé III - ciml.info
Tis is a set of introductory materials that covers most major aspects of modern machine learning (supervised and unsupervised learning, large margin methods, probabilistic modeling, etc.). It's focus is on broad applications with a rigorous backbone.
(21544 views)